The Computational Creativity and Archaeological Data project is working with the Antioch fieldbooks from the 1930’s. These fieldbooks have been scanned and are available for research here. This post will discuss the automated transcription of one of these fieldbooks.
Handwriting transcription is relatively new, but is mainstream and works well. For example, see Simon Willison’s blog. The Antioch fieldbooks offer an additional challenge for transcription, they are written in pencil which does not contrast well with the background color of paper. See the image below:
At first, when I used Azure Cognitive Services to transcribe text, the results were rather poor, as per below:
1 Juin. C'st un terrain situe a l' Est on la Renti ; inst contyn Le pek voyia à & maism ro'sine a fm muriau À la rent , pos ihr con Na Ih tivain - Jun 10m x3m
I rendered the images into black and white to increase the contrast. (Working code is here.) Below is a sample image and results.
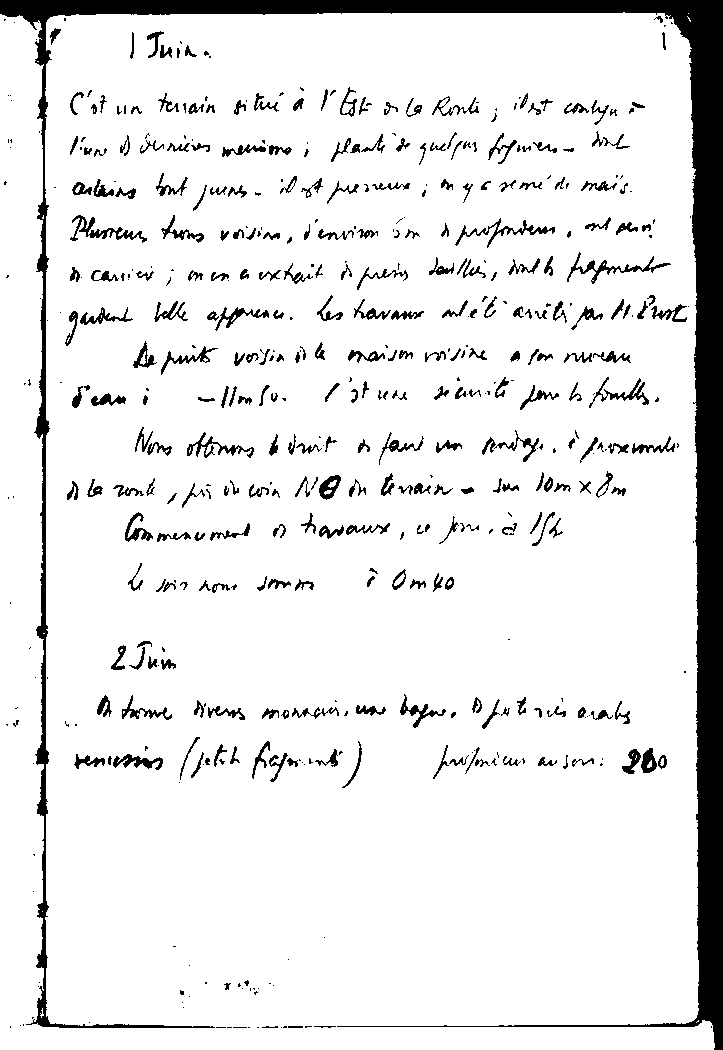
1 Juin. C'est un terrain situé à l'Est or la Route ; il est conlyn l'un 1 derrières massimo ; plante de quelpas figuiers - dal acting but jeers . it off presseurs ; in ya reme'de mai's. Plusieurs tuns voision, d'environ 5m de profondeur, as servi À carrier ; men a extrait is press dailles, with fragment gardent belle apparence. Les travaux net avéli pas 11. Pust De puits voisin A la maison voisine . for nouveau Sean : - 11mbo . I st use seemite love to fouls . None offirms & droit as faul un povagy, è prosimale À la conte , pi du coin NO in terrain - sun 10m xIm Commencement 1 travaux, 4 por, à Ifhe , Om40 2. Jun A frame Divers monacies , une bagno . A foto ries orals 4. remission ( patch fragments) profmeus au son : 200
As shown above, the results are better. I’d like to fine tune the image contrast process to improve results though.
Although the results improved, they are not that useful. I tried AWS Textract to improve results. For this page, here they are:
) Juin. C'st uin tanain situi a 1 Est is la Ronte ilst conlyn 1. Fun & burious menime; plants & guelpus togmen me anthoms but jurns it it preneure m y c remi'd mais Plusen two valian, jenvisor 5m A proformance and phri n carrier; men a extail is prems dailler, inth hagment gardent little appenence. he havane mit anition 11 Part L puils verying 1 a maison misine . for mean iean i - - limsu. / it use viamits from h fomells. Nons oftension i init to fani in andry. iproxemate 1 la wonte, , tris it win NO in train - in 10mx cm Commenument 1) havause, " pone. ' 15th le this home Jmm i Om40 2Jum time Avens moraan.un before. D fut in each renisms (ptob pro/mium wuson 260 ) Juin. C'st uin tanain situi a 1 Est is la Ronte ilst conlyn 1. Fun & burious menime; plants & guelpus togmen me anthoms but jurns it it preneure m y c remi'd mais Plusen two valian, jenvisor 5m A proformance and phri n carrier; men a extail is prems dailler, inth hagment gardent little appenence. he havane mit anition 11 Part L puils verying 1 a maison misine . for mean iean i - - limsu. / it use viamits from h fomells. Nons oftension i init to fani in andry. iproxemate 1 la wonte, , tris it win NO in train - in 10mx cm Commenument 1) havause, " pone. ' 15th le this home Jmm i Om40 2Jum time Avens moraan.un before. D fut in each renisms (ptob pro/mium wuson 260
AWS Textract translates more letters, but based on this one page, Azure Cognitive Services seems to better understand the French language text. AWS supports French, but I am not sure if there is a parameter to tell it to use French, like Azure Cognitive Services uses. Below is a program line for Azure Cognitive Services setting language = “fr”.
read_response = computervision_client.read(read_image_url, raw=True, model_version="2022-01-30-preview", language = "fr")
I also tried Google Cloud Platform (GCP). (I wrote about using GCP here.) GCP also supports French with “language_hints”:
image = vision.Image(content=content) response = client.text_detection( image=image, image_context={"language_hints": ["fr"]}, )
Below are the results for the page above:
-1 | Juina C'st un terrain situé à l'Est or La Ronti ; il est conly n l'une if Juniores mecione ; plante de quelqus figniers dink orting bout joins il y preveux ; yo semé de mais . Plusieur tous voisina , d'environ 5on à profondeus , ont pris ! gardend à carrier ; men a extrait is presies desitlers , wind to fragmento belle apparence . Les travaux atét anéli par 11. Pust te punts voisin is la maison voisine a for mureau -11m Su- / sture sécurité pour to fouilles . anday presente Nons oftenons uit os fami de la conte , fis in coin NO in terrain - fon 10m x 8m Commencement of travaux , ce jour , an Ist > Omko de Join home from 2. Jum tarme Divers monnaies , un reniesios ( jetch faforint ) m * bojne foteries orals . proponicur ausen : 200
Here are some additional results for you to judge: see this page and click the “next” link to browse further. This spreadsheet lists the pages and transcribed text in the “contrasted_pages” tab.